The security industry is undergoing an unprecedented transformation. Advances in artificial intelligence (AI) and machine learning (ML) are leading the way in a data-driven age, allowing us to solve complex problems and automate repetitive processes in ways that weren’t possible before. Just as important, AI is also helping us make sense of the ever-increasing amounts data we are using and generating.
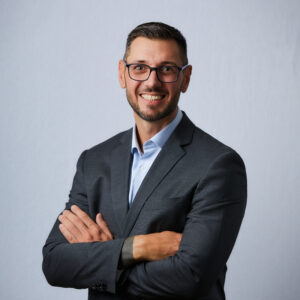
Almost every industry is finding new and innovative use cases for AI, and the world of security and surveillance is no exception. Modern surveillance systems generate a tremendous amount of data, especially when recording video for 24/7 operations, but most of this data goes unused. Capturing detailed footage over extended periods is essential for collecting evidence or making more informed decisions about incidents, in the past or in real-time, but finding what matters in a scene is extremely time-consuming, if not impossible, when done manually.
Why metadata matters
It is therefore essential to make valuable data more identifiable and actionable, which is where AI provides a solution. Using deep learning algorithms, AI collects and classifies information as metadata, which is then used to find, analyse, and act on the smallest details siphoned from thousands of hours of video footage. Metadata can comprise of anything from objects in a scene (such as cars, trucks, individuals, crowds, or even animals), object movement and direction, signs of intrusion or other types of actions, unique sounds (such as voices, alarms, or gunfire), and more specific details such as licence plates, faces, tracks, or the colour and model of vehicles.
Metadata gives context to every object and event in a scene, adding significantly more value to a surveillance system compared to a traditional surveillance system, which can only track basic details such as movement. This not only eliminates false positives, but also allows large amounts of recorded footage to be instantly collected, classified, searched, or retrieved for use. As a result, metadata can be used for real-time alarm triggering, post-event forensic searching, as well as statistical analysis and reporting.
AI-powered surveillance systems can also be integrated with other systems, such as GPS tracking, noise detection, environmental sensors, and point of sale transactional data, enabling broader applications and more unified insights. For this to be possible, however, a surveillance system needs to have an open API to ensure interoperability and seamless metadata integration.
AI surveillance at the edge
Data is always most valuable the moment it is generated, especially when being used for analytics. This is why AI analytics often run at the edge of a surveillance network, bringing the processing closer to the devices collecting the information and improving the accuracy of real-time analytics. Less latency between the origin of surveillance data and analytics allows for a timelier response to events. In a traffic scenario, for example, AI analytics at the edge can detect objects on the road or accidents and immediately activate signage to slow drivers or alert emergency services.
Another benefit of running analytics at the edge is that it allows for the highest quality video to be used for more detailed analysis. The traditional approach of running analytics on a distant server means that footage often needs to be compressed and degraded to minimise data traffic. By deploying analytics at the edge, rather than a centralised server, only the most relevant information needs to be sent across the network, which minimises network costs, bandwidth usage, and storage.
Smart surveillance for a safer future
When it comes to effective surveillance, the details matter. AI analytics offer us a unique opportunity to extract more meaningful information from surveillance. And with AI-enabled privacy masking, a permanent opaque mask can be applied to faces, number plates, or specific areas to uphold privacy laws and regulations.
There are also significant operational efficiencies that come with the advent of analytics, as businesses and governments can all use smart surveillance to make more informed, data-driven decisions. Private and public spaces can be made safer, traffic can be monitored to avoid congestion or prevent accidents, and even our natural environment can be safeguarded when coupled with environmental sensor analytics. By applying AI to the security industry, we can create a safer and smarter future for everyone.
Discussion about this post