In the pursuit of more effective transportation solutions, the challenge of managing traffic congestion and travel delays demands innovative approaches. The advent of synthetic data generation has opened new avenues for research and simulation, offering fresh perspectives on traffic patterns and infrastructure impacts. Recent advancements, particularly with solutions like SAS Data Maker, are poised to transform how we approach these issues.
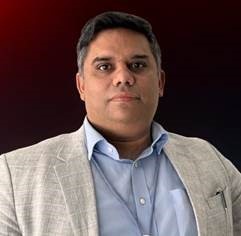
Navigating the Data Challenges in Transportation
Transportation research relies heavily on accurate data to model traffic patterns, assess the impact of infrastructure changes, and evaluate congestion mitigation strategies. Traditional data collection methods often struggle with limitations such as cost, time, and privacy concerns. As a result, researchers and policymakers are faced with the challenge of finding reliable, comprehensive data to guide their decisions.
SAS Data Maker is set to address these challenges by providing a state-of-the-art solution for synthetic data generation. Synthetic data, created through sophisticated algorithms rather than traditional data collection, mirrors the statistical properties and characteristics of real-world datasets. This approach not only ensures data privacy but also overcomes the limitations associated with conventional data-gathering methods.
The Impact of Synthetic Data Generation on Transportation Research
- Enhanced Simulation Accuracy: Synthetic data closely replicates real-world traffic conditions, enabling researchers to conduct more accurate simulations. This capability is crucial for testing various scenarios, such as optimising traffic signal timings or evaluating the effects of new transit routes.
- Efficiency and Cost Savings: Traditional data collection processes can be resource-intensive and time-consuming. Readily available synthetic datasets can streamline the research process, allowing researchers to focus on analysis and innovation rather than data acquisition.
- Privacy Protection: Privacy concerns are paramount when dealing with real-world travel data. Synthetic data maintains the integrity of privacy by providing realistic data without exposing sensitive information.
- Scalability and Flexibility: The ability to generate extensive volumes of synthetic data allows researchers to explore a wide range of traffic scenarios and test various hypotheses. This scalability enhances the depth and breadth of transportation research.
Looking ahead
Synthetic data is already making a significant impact across various sectors, including transportation. According to Gartner predictions, by 2026, 75% of businesses will use generative AI to create synthetic customer data, up from less than 5% in 2023. SAS experts illustrated the functionality of synthetic data generation at a recent, local Big Data Event, showing how it could be used to simulate mobile phone records for transportation research. This exercise highlighted the methodology for generating data, addressing challenges and post-processing required before data can be used.
SAS D[n]A Factory is similarly working around different use cases of synthetic data, creating innovative new applications to solve region- and industry-specific challenges whilst also improving overall profitability for businesses in the UAE.
Discussion about this post